SoS Musings #50 - Moving Automotive Cybersecurity into the Fast Lane
SoS Musings #50 -
Moving Automotive Cybersecurity into the Fast Lane
Connected and autonomous vehicles offer benefits, but they also introduce significant cybersecurity risks that can lead to the loss of life on the road. As vehicles increase in connectivity, they become more vulnerable to being hacked, manipulated, and disabled. Autonomous vehicles use Artificial Intelligence (AI), which applies Machine Learning (ML) algorithms to collect, analyze, and transfer data for decision-making. AI systems, like all IT systems, are vulnerable to attacks that could cause the vehicle to malfunction. The presence of vulnerabilities in such vehicles creates more opportunities for hackers to perform malicious activities. With connected and autonomous cars becoming increasingly part of our world, it is essential to address concerns regarding the cybersecurity of such vehicles through further research and solutions development.
There have been multiple studies that point out the vulnerabilities associated with modern connected vehicles that leave them open to hacking. Cybersecurity researchers analyzed nine different connected car Android apps designed to allow drivers to locate cars and unlock them via their smartphone, each of which was downloaded between 10,000 to one million times from the Google Play app store. They discovered that all nine of the mobile apps contained unencrypted usernames and passwords stored with the vehicle's Vehicle Identification Number (VIN), and in some instances, the car's license plate number in plaintext. Some of the apps were also found to be easily decompiled to read the app's code or actively save debugging data to the phone's SD card. As both the app and debugging code contain the user's username and password in plaintext, it would be easy for an attacker to steal these login details if they root the device or if malware is downloaded. Using the stolen credentials, they could log in to the app, unlock the user's car, and steal it. With access to the app, the attack could also start the vehicle's engine remotely. In another discovery, researchers with the cybersecurity firm, Trend Micro, revealed a cybersecurity issue in the Controller Area Network (CAN) protocol, which connects car components, such as parking sensors, the infotainment system, the airbag, and the active safety system, enabling them to communicate and send commands to each other within the car's network. The presented proof-of-concept attack abuses a feature in which a device in a car goes into Bus Off state, where it is cut off from the CAN and prevented from reading or writing any data onto the CAN when it sends out too many errors. The purpose of this feature is to isolate malfunctioning devices and to stop them from triggering other modules and systems on the CAN. The Trend Micro researchers' attack is considered a Denial-of-Service attack in that it triggers this specific feature by inducing enough errors so that a targeted device or system on the CAN is put into Bus Off state and rendered inoperable, thus potentially leading to the deactivation of airbags, anti-lock brakes, and other essential vehicle components. Research from Carnegie Mellon University's CyLab led to the discovery of a new class of cybersecurity vulnerabilities in connected vehicles. According to the CyLab researchers, these vulnerabilities could enable attackers to circumvent a vehicle's Intrusion Detection System (IDS) and shut down different car components, including the engine, through the execution of carefully crafted computer code from a remote location. They stressed that the exploitation of the new class of vulnerabilities does not require threat actors to manipulate hardware or physically access the targeted vehicles. These findings further emphasize the repercussions of weak automobile cybersecurity and the importance of increasing such research to inform automakers on the implementation of security in the design of vehicles and their systems.
Studies have revealed the weakness of AI systems used by autonomous vehicles to model hacking, also known as adversarial ML. A report by the European Union Agency for Cybersecurity (ENISA) and the Joint Research Center (JRC) titled "Cybersecurity Challenges in the Uptake of Artificial Intelligence in Autonomous Driving," delved into potential attacks aimed at interfering with an autonomous vehicle's AI system and disrupting safety-critical functions. Some examples of these attacks outlined in the report include adding paint on the road to misguide navigation and placing stickers on a stop sign to prevent recognition. These modifications can result in the misclassification of objects by the vehicle's AI system. In order to perceive the world, autonomous cars rely on multiple sensors. Most autonomous vehicle systems use a mix of cameras, radar sensors, and LiDAR (Light Detection and Ranging) sensors through which data is combined by an on-board computer to create a complete view of the car's surroundings. This data allows the vehicle to navigate the world safely. Although the implementation of multiple sensor systems enhances the functionality and safety of vehicles, they have been found to be vulnerable to attacks. A study by researchers from the University of Washington, the University of Michigan, Stony Brook University, and the University of California, Berkeley showed that it is possible to fool the ML algorithms used in camera-based perception systems into misinterpreting stop signs as speed limit signs and turn signs as stop signs by making slight changes to the street signs, such as adding stickers and camouflage graffiti. In another study conducted by the RobustNet Research Group at the University of Michigan, researchers demonstrated the possibility of tricking a vehicle's LiDAR-based perception system into seeing a nonexistent obstacle, like another car, by spoofing the LiDAR sensor signals and fooling the ML model, used to process the sensors' data. The researchers demonstrated two LiDAR spoofing attacks on an autonomous driving system widely used among carmakers, called Baidu Apollo. In the first attack, they showed how an attacker could suddenly stop a moving vehicle by tricking it into thinking an obstacle has appeared in its path. In the second attack, they used a spoofed obstacle to fool the vehicle that had been halted at a red light to remain stopped after the light turns green. McAfee researchers further emphasized the weakness of ML systems used in autonomous vehicles by tricking a Tesla vehicle into speeding up. They did this by placing a piece of electrical tape horizontally across the middle of the "3" on a 35 MPH speed limit sign. This change caused the vehicle to read the 35 MPH sign as 85 MPH and its cruise control system to automatically accelerate. These studies call for more attention on cybersecurity in regard to the use of AI in autonomous driving.
Cisco released an open-source hardware tool called 4CAN with accompanying software that can help automobile researchers and car manufacturers identify potential vulnerabilities in their on-board computers. 4CAN was designed to fuzz test vehicle components to identify vulnerabilities, explore the CAN commands used to control and interact with the vehicle, validate communication policy for intra-CAN bus communication, and more. In collaboration with the connected and autonomous vehicle testing facility Mcity, researchers at the University of Michigan introduced a cybersecurity tool called the Mcity Threat Identification Model, which could help academic and industry researchers examine the probability and severity of potential threats facing autonomous vehicles. This tool provides a framework that considers attacker skill levels, attack motivations, vulnerable vehicle system components, attack methods, and the impact of such attacks regarding privacy, safety, and financial loss. A framework developed by researchers at the University of Texas, San Antonio (UTSA) aims to help determine what and where vulnerabilities in connected and autonomous cars can be exploited. Using this framework, the team tried to develop and apply cybersecurity authorization policies in various access control decision points to prevent cyberattacks and unauthorized access to sensors and data in these vehicles. The U.S. Department of Transportation's National Highway Traffic Safety Administration (NHTSA) also released guidance to the automotive industry for improving modern vehicle cybersecurity, calling on automakers to share more information about cybersecurity risks with each other, implement additional vulnerability reporting/disclosure programs, perform risk assessment, conduct penetration tests, and more. Such efforts must continue to reveal and address vulnerabilities in connected and autonomous vehicles, as well as spark the development of solutions to combat cybersecurity threats facing such vehicles.
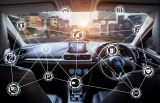